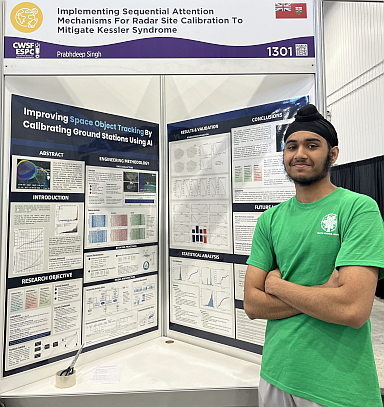
Prabhdeep Singh
Implementing Sequential Attention Mechanisms For Radar Site Calibration To Mitigate Kessler Syndrome
London Central Secondary School
Since 2010, the quantity of objects in Low Earth Orbit (LEO) has doubled, precipitating a surge in the probability of collisions. These collisions not only inflict damage on functional satellites but also spawn additional space debris. Traveling at speeds of tens of kilometers per second, these space debris have the potential to cause more debris and subsequent collisions, ultimately filling earths orbit. This scenario risks rendering LEO impassable, curtailing space exploration and research. Present space surveillance relies on radar sites, producing probabilistic collision assessments, which in turn endanger both the safety and operational integrity of pivotal space infrastructure. This project introduces a deep learning model to calibrate multi-station data, enhancing the precision of space object tracking. This system promises to considerably reduce the risk of collisions in LEO, thereby safeguarding the sustainability and security of space activities.